A Framework for Automated Measurement of Responsible AI Harms in Generative AI Applications
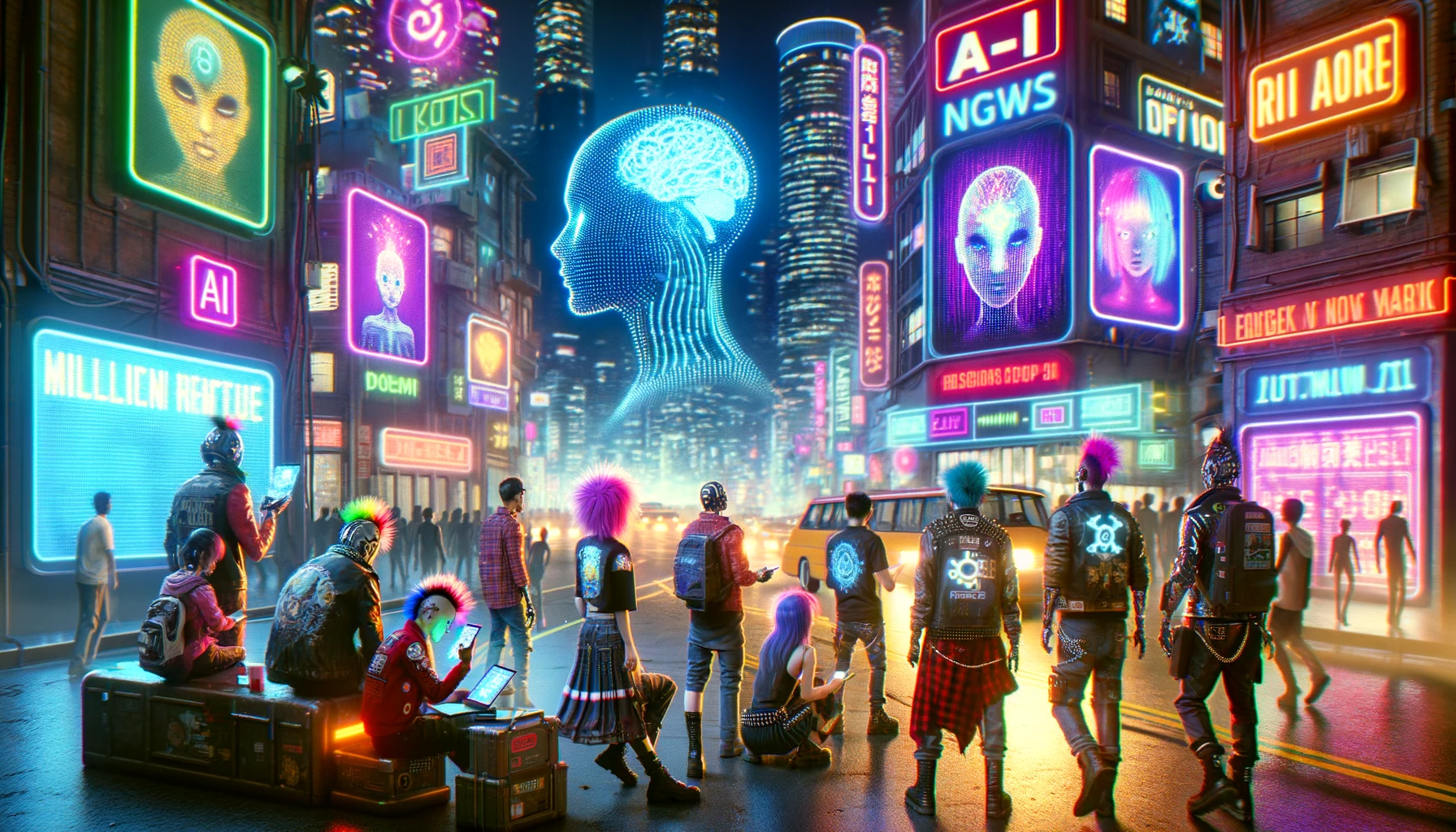
## Purpose
The paper presents a framework for automated measurement of responsible AI (RAI) metrics in large language models (LLMs), focusing on identifying and evaluating potential harms caused by these models.
## Methods
- **Data Generation**: Using templates and parameters to simulate user-AI interactions in various scenarios, mimicking real-world use of LLMs.
- **Evaluation**: Applying annotation guidelines to assess LLM-generated content for potential harms, both quantitatively and qualitatively.
## Key Findings
1. **Automated Harm Measurement**: The framework allows for automated, scalable harm measurement in LLMs.
2. **Use of GPT-4**: The utilization of GPT-4 for evaluating other LLMs in terms of harm generation.
3. **Experimental Design Efficacy**: The framework's efficacy demonstrated through experiments evaluating different LLMs for potential harm generation.
4. **Comparison of Models**: Insights into the relative performance of different LLMs regarding responsible AI principles.
## Discussion
This research is pivotal in advancing the responsible use of LLMs. It addresses the need for scalable and automated methods to measure potential harms, which is essential given the rapid development and deployment of these models.
## Critiques
1. **LLM-based Evaluation Risks**: The inherent risks in using LLMs for evaluating other LLMs, especially considering their propensity to generate harmful content.
2. **Data Generation Validity**: Concerns about the ecological validity of the data generated through simulated user interactions.
3. **Measurement Resource Development**: The challenges in developing reliable and valid measurement resources for assessing harms.
## Tags
#ResponsibleAI #LargeLanguageModels #AutomatedMeasurement #AIHarms #AIAlignment #GPT4 #AIethics #EvaluationFramework