AI Alignment and Social Choice: Fundamental Limitations and Policy Implications
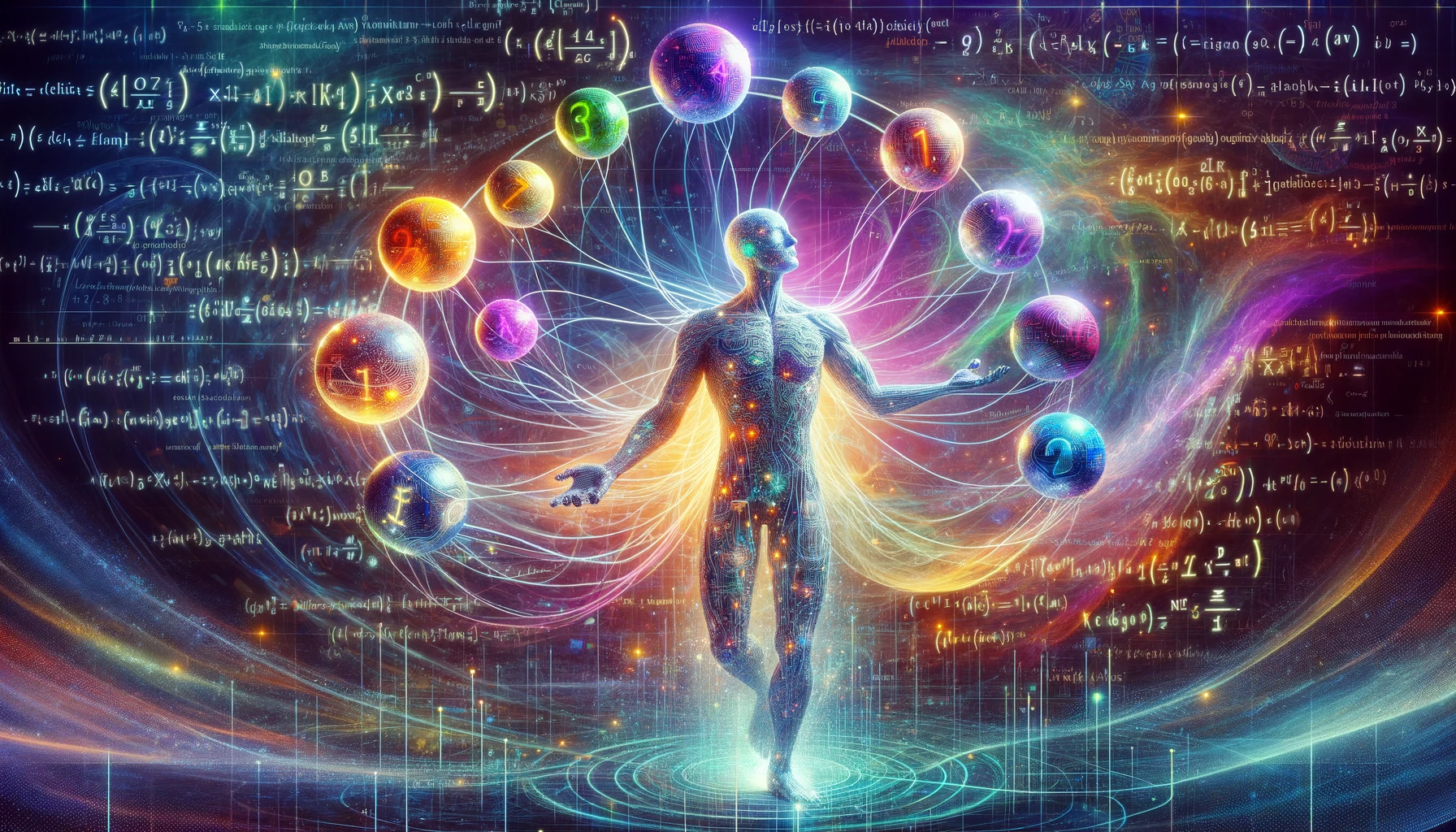
The paper: http://arxiv.org/abs/2310.16048
## Purpose
The paper addresses the challenge of aligning AI agents with human intentions and values, focusing on the limitations of Reinforcement Learning with Human Feedback (RLHF) in the context of democratic norms and social choice theory.
## Methods
- Exploration of RLHF and its application in aligning AI agents.
- Analysis of Arrow-Sen impossibility theorems within the context of RLHF.
- Examination of the implications for AI governance and policy.
## Key Findings
1. No unique voting protocol can universally align AI systems using RLHF through democratic processes.
2. Aligning AI agents with the values of all individuals violates certain private ethical preferences.
3. Transparent voting rules are needed for model builder accountability.
4. Focus should be on developing AI agents narrowly aligned to specific user groups.
5. Universal AI alignment using RLHF is impossible.
## Discussion
The findings highlight a significant challenge in AI alignment, emphasizing the complexity of integrating human ethics and values in AI systems democratically. The research underscores the need for transparency in AI governance and the impracticality of achieving universal AI alignment.
## Critiques
1. The paper could benefit from more diverse perspectives on AI alignment beyond RLHF.
2. There's a need for empirical evidence supporting the theoretical claims.
3. The paper may oversimplify the complexity of human values and ethical considerations.
## Tags
#AIAlignment #SocialChoiceTheory #RLHF #AIethics #AIgovernance.