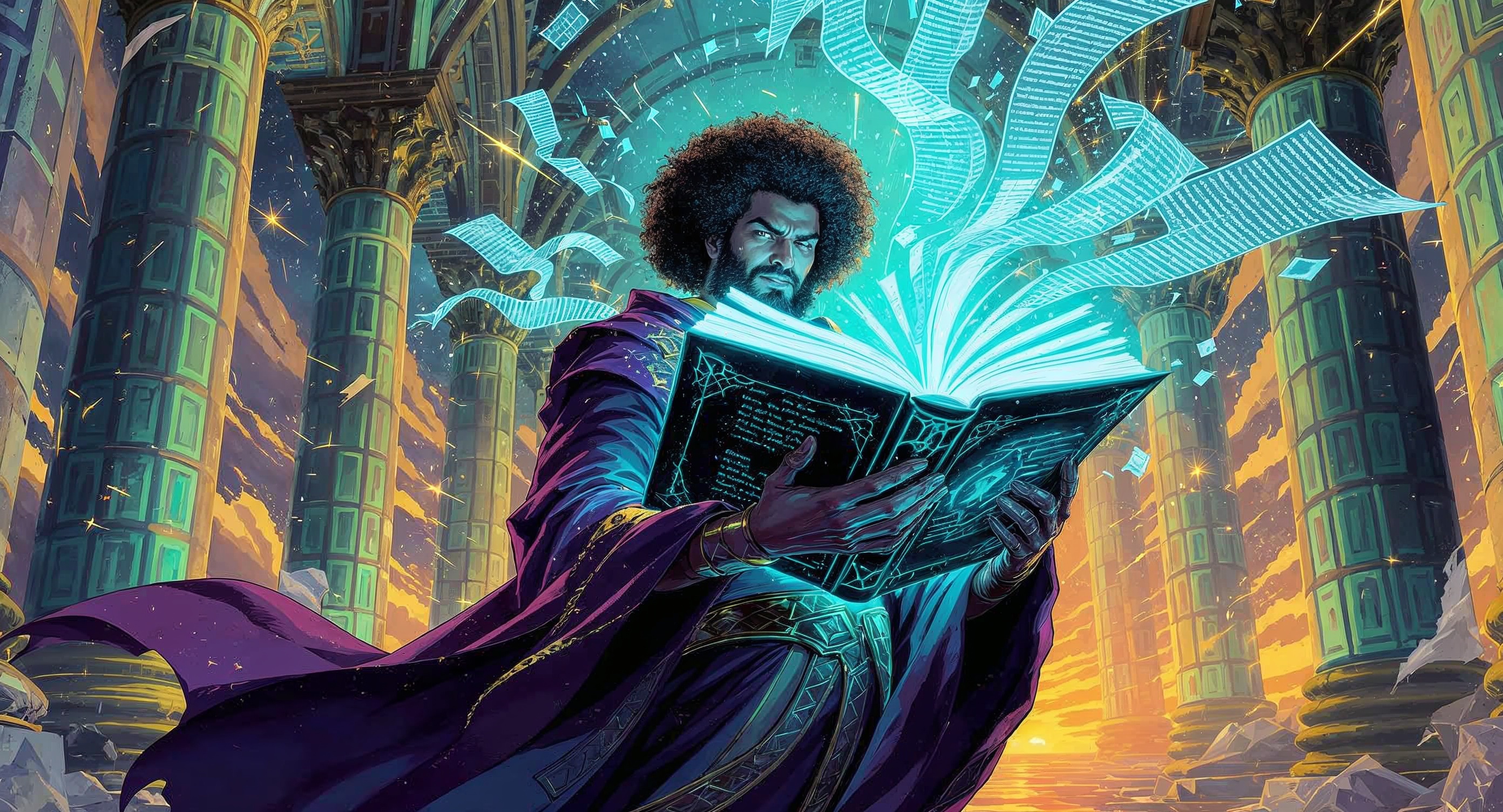
Analyzing the Influence of Language Model-Generated Responses in Mitigating Hate Speech on Social Media Directed at Ukrainian Refugees in Poland
The paper: http://arxiv.org/abs/2311.16905
## Purpose
The study "Analyzing the Influence of Language Model-Generated Responses in Mitigating Hate Speech on Social Media Directed at Ukrainian Refugees in Poland" investigates the potential of using responses generated by Large Language Models (LLMs), supplemented with verified knowledge links, to counteract hate speech and polarization on social media.
## Methods
- Employing LLMs to generate responses to harmful tweets.
- Fine-tuning a detection model for harmful tweets, combining OpenAI embeddings with logistic regression.
- Utilizing a two-stage approach for intervention: identifying relevant verified news articles and generating a neutralizing message based on this content.
- Conducting an A/B test on Twitter to compare the impact of model-generated responses versus control conditions.
- Statistical analysis of the impact on user engagement, sentiment, and replies.
## Key Findings
1. LLM-generated responses significantly diminish user engagement with harmful tweets.
2. The engagement of users with harmful tweets reduces by over 20% when responded to with model-generated content.
3. The ratio of replies to a harmful tweet increases, especially when the original tweet is not a reply itself.
4. The overall sentiment of tweets in discussions is not significantly altered by the intervention.
5. The introduction of verified links in responses leads to a greater increase in the number of replies to a harmful tweet.
## Discussion
This research is relevant to the field of AI ethics and online community management, demonstrating the efficacy of AI-generated responses in mitigating hate speech on social media. It highlights the potential of using AI as a tool for social good, particularly in moderating online discourse and reducing the spread of harmful content.
## Critiques
1. Limited generalizability due to the specific focus on Ukrainian refugees in Poland.
2. Potential bias in the detection model that could affect the classification of tweets as harmful.
3. The need for manual verification of model-generated responses, which might limit scalability.
4. The study does not conclusively prove changes in public sentiment, necessitating further research.
5. Reliance on external sources for fact-checking, raising questions about the sustainability and adaptability of the model.
## Tags
#AI #HateSpeech #SocialMedia #LanguageModels #EthicsInAI #OnlineModeration.