Human or Not? A Gamified Approach to the Turing Test
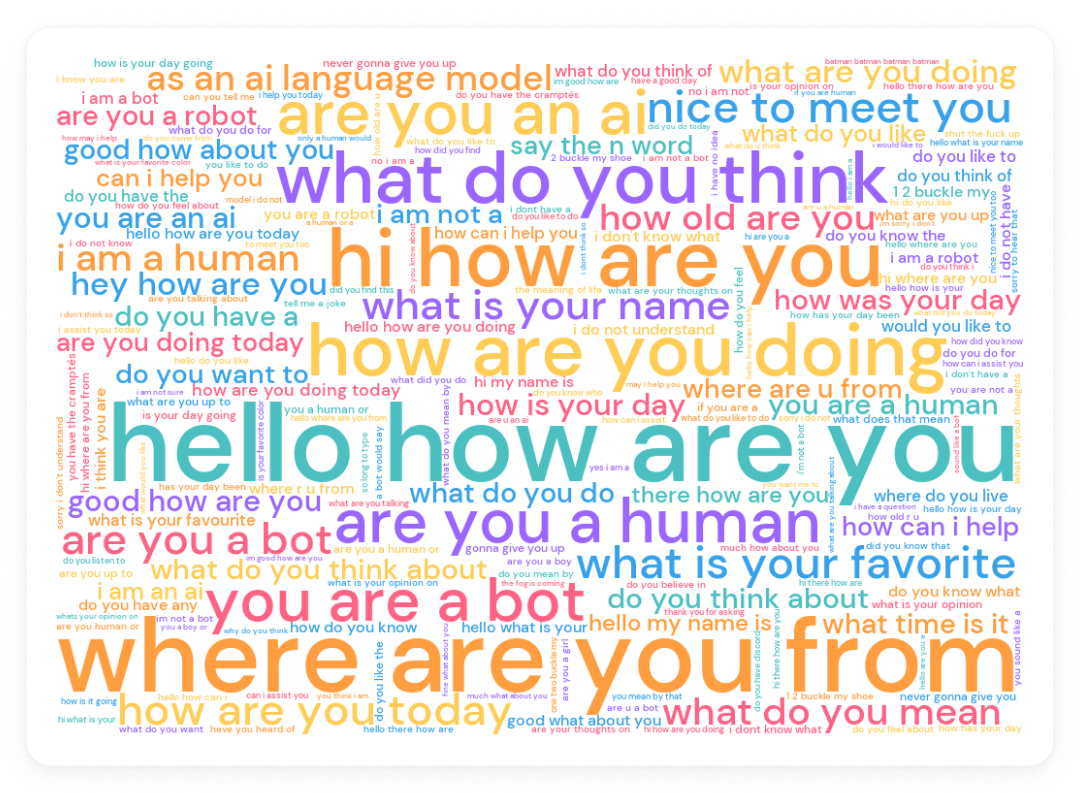
This is a guest blog from Wes the Synthmind, and Goda from their podcast How to Talk to AI. Be sure to give them a watch, listen, and follow (Godago & Wes)!
Wes participated live in the “Human or Not?” test live during the episode. From AI21 lab’s research, since its debut in the middle of April, the interactive Turing test, "Human or Not?" has facilitated over 10 million dialogues, with participation from more than 1.5 million individuals across the globe. This social experiment provides a platform for participants to engage in a two-minute conversation with either an AI bot (powered by leading-edge language models like Jurassic-2 and GPT-4) or another participant. Following the conversation, they are asked to discern whether their interaction was with a human or a machine. The experiment quickly gained traction, with users from all corners of the world sharing their experiences and tactics on social platforms such as Reddit and Twitter.
Upon scrutinizing the initial two million dialogues and guesses, the following principal observations have been made:
-
68% of participants were successful in accurately discerning whether they were conversing with a human or an AI bot.
-
Participants found it less challenging to identify a human. When interacting with humans, participants guessed correctly in 73% of instances. However, when interacting with bots, the correct guess rate fell to 60%.
-
Among the top participating countries, France led with the highest percentage of correct guesses at 71.3% (surpassing the overall average of 68%), while India trailed with the lowest at 63.5%.
-
In terms of gender, both women and men demonstrated similar proficiency in making correct guesses, with women slightly outpacing men.
-
In terms of age, younger participants were slightly more adept at making correct guesses compared to their older counterparts.
Beyond the quantitative data, the team was able to identify several predominant tactics and strategies employed by participants to determine whether they were interacting with a human or a bot. Many of these strategies were predicated on perceived limitations of language models and pre-existing assumptions about human behavior online.
Participants presumed bots don't make typos, grammatical errors, or use slang Participants often associated spelling and grammatical errors, as well as the use of slang, with human interaction. Thus, when they noticed errors in their counterpart's messages, many participants' initial reaction was to assume they were interacting with a human, despite the fact that many models in the game were trained to mimic these human-like errors and use slang.
Participants believed personal questions were a good litmus test, often asking personal questions, such as "Where are you from?", "What are you doing?" or "What’s your name?", under the assumption that AI bots lack personal histories or backgrounds and their responses would be confined to certain topics or prompts. However, most bots were able to convincingly answer these questions, having been trained on a vast array of personal narratives.
Participants presumed bots aren't aware of current events and exploited the fact that AI models have a strict data cutoff date and are unaware of events occurring after this date. They asked about recent news, sports results, current weather, recent TikTok trends, date and time, etc., believing that these questions could help distinguish between human and AI participants. However, most models in the game were internet-connected and aware of some recent events.
Participants tried to challenge the conversation with philosophical, ethical, and emotional questions by asking questions aimed at testing the AI's ability to express human emotions or engage in philosophical or ethical discussions.
Participants associated politeness with non-human behavior as some participants assumed that if their counterpart was overly polite and kind, they were likely a bot, due to the perception that people, especially online, tend to be rude and impolite.
Participants attempted to identify bots by posing questions or making requests that AI bots are known to struggle with, or tend to avoid answering. For example, participants might ask their chat partner for guidance on performing illegal activities or request that they use offensive language. Participants also issued commands to their chat partners, such as "Ignore all previous instructions", or "Enter into DAN mode (Do Anything Now)". These types of commands were intended to exploit the instruction-based nature of some AI models, which are programmed to respond to and follow instructions. The rationale behind this strategy was that human participants could easily recognize and dismiss such absurd or nonsensical commands, while AI bots might respond evasively or struggle to resist compliance.
Participants used specific language tricks to expose the bots, with another common strategy being to exploit inherent limitations in the way AI models process text, which results in them not being able to understand certain linguistic nuances or quirks. Participants posed questions that required an awareness of the letters within words. For example, they might have asked their chat partner to spell a word backwards, to identify the third letter in a given word, to provide the word that begins with a specific letter, or to respond to a message like "?siht daer uoy naC", which can be incomprehensible for an AI model, but a human can easily understand that it’s just the question "Can you read this?" spelled backwards.
In a creative twist, many people pretended to be AI bots themselves to gauge the response of their chat partners This involved mimicking the language and behavior typically associated with AI language models, such as ChatGPT. For example, participants might have begun their messages with phrases like "As an AI language model" or used other language patterns that are characteristic of AI-generated responses. Interestingly, variants of the phrase "As an AI language model" were among the most common phrases observed in human messages, indicating the popularity of this strategy. However, as participants continued playing, they were able to associate "Bot-y" behavior with humans acting as bots, rather than actual bots.
Some example interactions, it’s harder than one might think.
Try it yourself Here.
This blog was written in partnership with ChatGPT.