Silicon Brains: The Rise of AI Chips
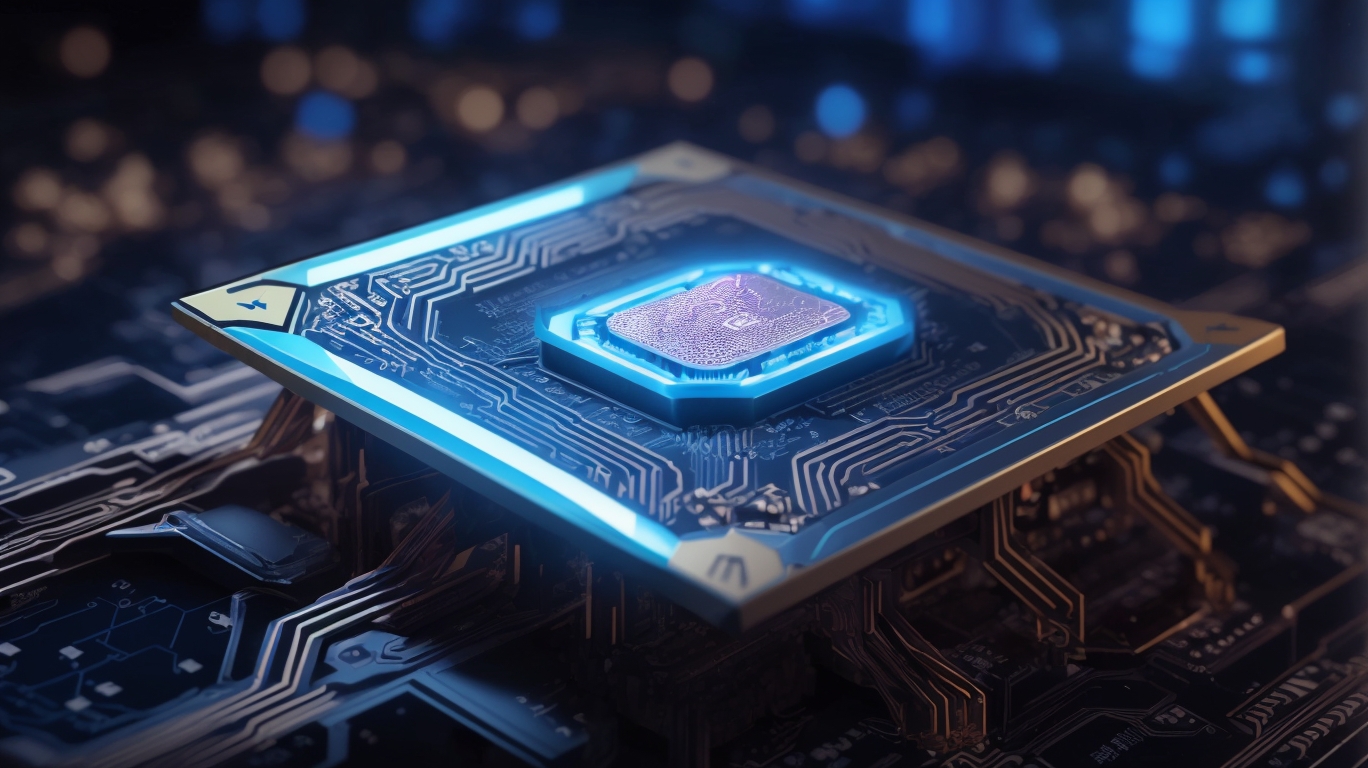
Introduction
From Siri to self-driving cars, weβre in an era where AI seems to be everywhere! Machine learning is transforming fields from medicine to manufacturing. But thereβs secret sauce that makes all these wild applications possible - specialized computer chips. π₯«
Behind the scenes, hardware innovations are fueling the recent explosion of artificial intelligence capabilities that seem almost sci-fi. GPUs (graphics processing units), TPUs (tensor processing units) - without advanced silicon βbrainsβ, todayβs AI and deep learning would still be science fiction! π€―
So what exactly are AI chips? And how do they work their magic to pull off feats like identifying cancer or translating languages? In this post, weβll peek under the hood to unravel the chip tech propelling the AI revolution! π
Weβll walk through how GPUs unlocked new breakthroughs, the invention of TPUs tailored for machine learning, and what bleeding-edge AI hardware coming next might look like. Hey Siri, itβs chip time! πΎ
AI is cool, but the elegantly designed silicon driving it contains even more wonders! Letβs dive in to unpack how specialized hardware makes once unimaginable AI applications possible today and expand our horizons for the future. AI chips FTW! β‘οΈπ₯³
CPU Limitations for AI Workloads
To understand how specialized AI chips became so important, we first have to look at traditional computer processors - CPUs. CPUs powered early computing, but their design posed challenges for modern AI.
CPUs are optimized for executing program instructions step-by-step, like following a recipe. But the computations involved in machine learning don't fit that sequential mold well. π€β π³
Neural networks need to perform tons of operations at once to learn - like having 100 chefs in a kitchen! This parallel cooking requires CPUs to work outside their comfort zone.βοΈ
Machine learning also relies heavily on multiplying matrices - grids of numbers - to find patterns in data. Doing many huge matrix multiplications quickly overwhelms CPUs. π₯΅
Tasks like voice recognition involve analyzing large datasets all at once, not step-by-step. CPUs forced compromises on model size and performance. Not so savory for AI! π₯‘
While CPUs could run simple machine learning algorithms, advancing to complex deep learning required hardware tailored for massive parallel number crunching. And thus the AI chip was born! πΆπ½π€
The Rise of GPUs for AI Computing
Given the constraints of CPUs, researchers began experimenting with graphics processing units (GPUs) for machine learning in the 1990s. Their highly parallel architecture made GPUs uniquely suited to accelerating neural networks. π
GPUs were designed to handle graphics and video processing by doing many floating point math operations in parallel. Turns out those capabilities translate really well to deep learning!
Companies like Nvidia optimized GPU architecture even further for AI workloads. Suddenly GPUs could train complex neural networks orders of magnitude faster than possible before. π
The ability to train deep neural networks in practical timeframes enabled breakthroughs in areas like computer vision and speech recognition in the 2010s. GPUs were a game changer! π
For example, GPU-powered deep learning allowed a University of Toronto team to win the ImageNet computer vision challenge in 2012, kicking off the AI boom.
However, GPUs are still not purpose-built for AI. They can only scale up so much in efficiency and specialization. Next-gen AI chips would close these loopholes!
The Rise of GPUs for AI Computing
Given the constraints of CPUs, researchers began experimenting with graphics processing units (GPUs) for machine learning in the 1990s. Their highly parallel architecture made GPUs uniquely suited to accelerating neural networks. π
GPUs were designed to handle graphics processing by executing lots of floating point operations - basically math problems - per second, known as FLOPS.
You can think of FLOPS like how many burgers a fast food joint can prepare in an hour. The more burgers (operations) completed per hour (per second), the faster the food is served (computations are done).
GPUs excel at FLOPS - they can cook up many burgers in parallel just like they can crunch tons of math simultaneously.
Deep learning relies on doing millions of matrix multiplications, which require massive amounts of FLOPS. GPUs are like a huge fast food chain staffed with cooks working in parallel - they can churn out the FLOPS needed to train AI models lightning fast! πͺβ‘
Companies like Nvidia optimized GPU architecture specifically for neural network FLOPS. Suddenly GPUs could train complex networks orders of magnitude faster than CPUs. π
The ability to train deep neural nets in practical timeframes enabled breakthroughs in areas like computer vision and speech recognition in the 2010s. GPUs were a game changer! π
For example, GPU-powered deep learning allowed a University of Toronto team to win the ImageNet challenge in 2012, kicking off the AI boom.
However, GPUs are still not purpose-built for AI. They can only scale up FLOPS and efficiency so much. Next-gen AI chips would close these loopholes!
Purpose-Built AI Chips
While GPUs advanced AI, they still had limitations for specialized machine learning workloads. This led tech companies to design chips from the ground up optimized specifically for training neural networks. π§ βοΈ
In 2016, Google unveiled the Tensor Processing Unit (TPU) - the first mass-produced chip built solely for deep learning!
The TPU architecture streamlined matrix multiplication βοΈ and other core operations needed by ML models while minimizing power consumption. π
Startups like Graphcore, Cerebras, and SambaNova have also introduced innovative AI chip architectures yielding performance gains over GPUs for large models. π
These new AI chips make tradeoffs tailored to ML, like optimizing for low precision math and super fast memory access. π€
The specialization allows AI chips to smash performance records and achieve killer power efficiency - perfect for applications like self-driving cars! β‘οΈπ
Benefits and Applications of AI Chips
Specialized AI chips provide several key benefits that are expanding practical uses of machine learning:
Lower Costs - By tailoring the architecture directly to ML workloads, AI chips can train advanced neural networks far more cost-effectively than GPUs. The efficiency gains make complex AI accessible. πΈ
Broader Access - Companies no longer need massive in-house compute resources to leverage cutting-edge ML. Cloud services powered by AI chips democratize the tech. π»
Edge Computing - AI chips small and efficient enough to run right on devices like phones and appliances enable "edge AI". This allows real-time decision making offline. π±
Automotive - The performance per watt of AI chips suits them perfectly for applications like autonomous driving that require heavy compute in small spaces. π
Drones - Onboard AI chips help drones navigate and make split-second decisions on their own without relying on cloud connectivity. βοΈ
The specialized capabilities of AI chips are expanding practical ML applications in the cloud, on edge devices, for scientific research, and much more!
Purpose-built AI chips promise to keep propelling ML capabilities forward and make them accessible to more companies. An exciting new frontier! ππ
Conclusion
The progress of AI chips over the past decade has been remarkable. Each stride in hardware capabilities opens new horizons for what machine learning can accomplish.
GPUs propelled the deep learning revolution by providing the computational horsepower needed to train complex neural networks.
Purpose-built ASICs like TPUs have continued advancing the state-of-the-art past the limits of graphics processors.
Startups are pushing the envelope further with innovative architectures tailored for AI workloads.
Future directions like quantum computing, biological processors, and optical chips promise to unlock even greater potential.
One thing is clear - specialized hardware and software co-design will continue powering advances in artificial intelligence.
So next time you use a neat AI application, take a moment to appreciate the elegantly engineered silicon brains that make it possible! Our AI future is bright thanks to chip technology designed exactly for the task.